Deep Learning, Part 2
As I had mentioned, Deep Learning is primarily a form of Unsupervised Learning. However, it differs from classic Unsupervised Learning algorithms in two ways. Instead of using a small (2 to 10) number of large clusters, Deep Learning flips the learning process around and looks for a large number (10,000 - 500,000) of small clusters (Overcompleteness). This is a computationally much more expensive procedure, which is why it has only started becoming viable in the past 10 years.
Second, most classic Unsupervised Learning makes the assumption that every piece of input is of equal importance. In terms of a task like Face Recognition, this clearly is not a correct assumption as pixels on the face is much more important than pixels in the background. Deep Learning introduce a Sparsity term that allows the algorithm to decide for itself which pixels are more important, and set unimportant pixels to have zero weightage.
Once the large set of clusters are found, we basically have a set of centroid points were the datapoints are densest. In terms of Face Recognition, the cluster centroids are where most of the faces reside. We can now use these centroids as features. This is achieved by expressing the original datapoint (eg, an image's raw pixel values) in terms of "distance" from the centroids. Once again a Sparsity term is used to ensure that some centroids are more important than other centroids (ie, distant centroids have zero weightage). This ensures that the original datapoint is expressed in terms of only a small number of nearby centroids.
This is essentially what happens in one single layer of the Deep Learning feature transformation process. By stacking multiple Deep Learning layers, the centroids that get selected in each layer become increasingly complex. Since Deep Learning only transform the original datapoint into a different set of features, we stack a a Supervised Learning algorithm such as Support Vector Machine on top to perform the actual task of classification.
As I had mentioned, Deep Learning is primarily a form of Unsupervised Learning. However, it differs from classic Unsupervised Learning algorithms in two ways. Instead of using a small (2 to 10) number of large clusters, Deep Learning flips the learning process around and looks for a large number (10,000 - 500,000) of small clusters (Overcompleteness). This is a computationally much more expensive procedure, which is why it has only started becoming viable in the past 10 years.
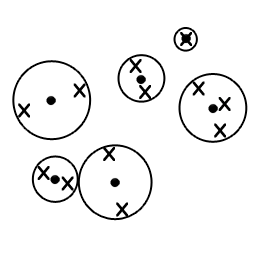
Second, most classic Unsupervised Learning makes the assumption that every piece of input is of equal importance. In terms of a task like Face Recognition, this clearly is not a correct assumption as pixels on the face is much more important than pixels in the background. Deep Learning introduce a Sparsity term that allows the algorithm to decide for itself which pixels are more important, and set unimportant pixels to have zero weightage.
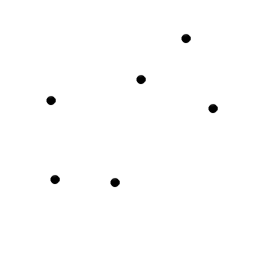
Once the large set of clusters are found, we basically have a set of centroid points were the datapoints are densest. In terms of Face Recognition, the cluster centroids are where most of the faces reside. We can now use these centroids as features. This is achieved by expressing the original datapoint (eg, an image's raw pixel values) in terms of "distance" from the centroids. Once again a Sparsity term is used to ensure that some centroids are more important than other centroids (ie, distant centroids have zero weightage). This ensures that the original datapoint is expressed in terms of only a small number of nearby centroids.
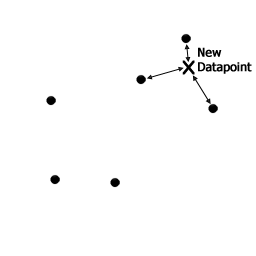
This is essentially what happens in one single layer of the Deep Learning feature transformation process. By stacking multiple Deep Learning layers, the centroids that get selected in each layer become increasingly complex. Since Deep Learning only transform the original datapoint into a different set of features, we stack a a Supervised Learning algorithm such as Support Vector Machine on top to perform the actual task of classification.
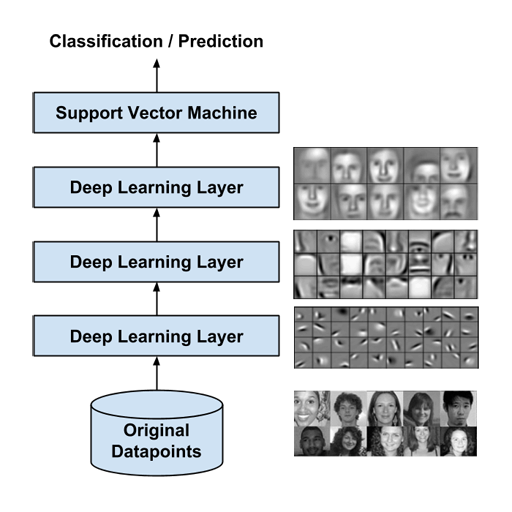
Last edited: